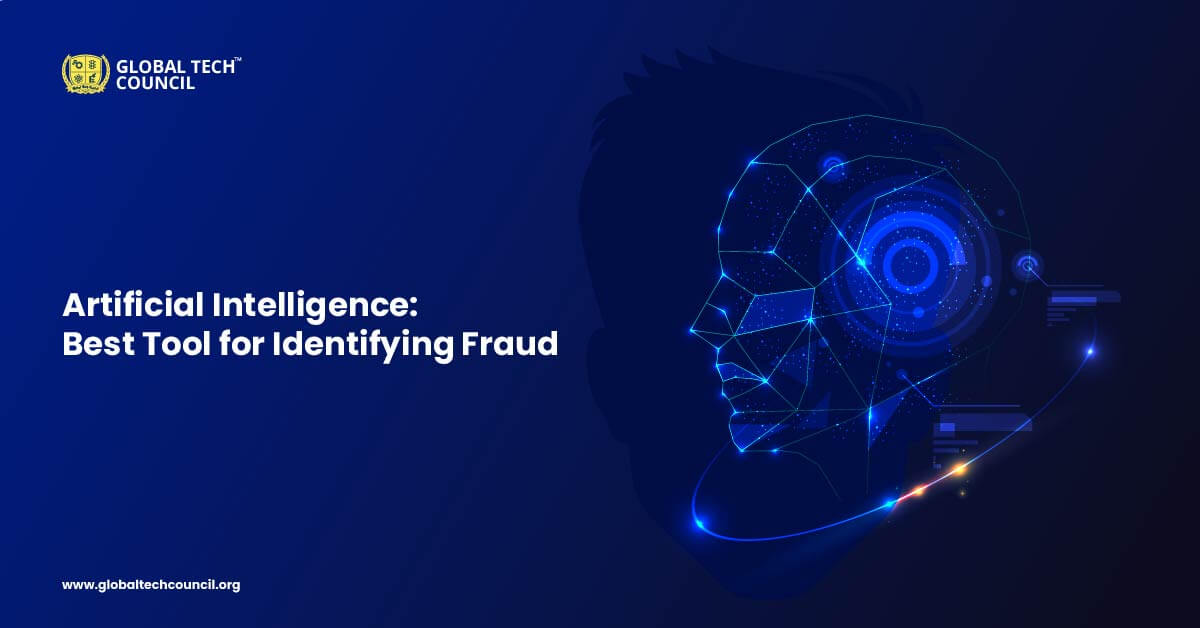
Fraud detection is a great application for machine learning, with a proven track record in industries like banking and insurance.
For those confused between artificial intelligence and machine learning; machine learning refers to analytic methodologies that understand patterns in datasets without any human analyst intervention. Whereas, artificial intelligence is a much broader concept. AI deploys specific types of analytics to accomplish tasks like identifying a fraudulent transaction or controlling a driverless vehicle.
Consider machine learning to be a technique for developing analytical models, and AI to be the use of such models.
Fraud cost the world economy £3.2 trillion in 2018, according to Infosecurity Magazine. Fraud losses can amount to more than 10% of a company’s entire spending in rare cases. Such large losses force businesses to look for innovative ways to prevent, detect, and eradicate fraud. The most potential technical weapon in the battle against financial fraud is machine learning.
What is the definition of fraud detection?
The term “fraud” is defined as “the crime of misleading others in order to get money.” And to be precise, it’s nothing new only the ways of committing fraud have evolved with time. Since people began exchanging commodities and services, there has always been a risk of one side defrauding the other. And there’s always been the possibility that a third party may defraud both the vendor and the customer.
Fraud has taken on new forms as e-commerce has grown in popularity, and it is now more potent than ever. Scam artists enjoy the benefits of every weak spot in any system they can uncover as the volume of e-commerce, online banking, and online insurance grows.
Sensitive data is frequently stolen, and millions of dollars are wasted before specialists can repair a system. On a worldwide scale, fraud has become a serious concern and an uncontrollable expense for e-commerce firms.
The e-commerce and banking businesses are currently preoccupied with preventing, detecting, and eradicating fraud. Machine learning development services are one of the most promising ways to achieve them.
Machine Learning-based fraud detection is achievable thanks to ML algorithms’ capacity to learn from previous fraud trends and spot them in future transactions. When it comes to the speed with which information is processed, machine learning algorithms appear to be more effective than people. In addition, machine learning algorithms may detect complex fraud qualities that a person cannot.
AI-based fraud detection and prevention strategies
Machine learning for fraud detection is based on the idea that fraudulent transactions have distinct characteristics that legal transactions do not. Machine learning algorithms recognize patterns in financial transactions and determine whether a transaction is valid based on this assumption. Machine learning fraud detection systems outperform humans by a wide margin.
Some of the strategies are:
Creating Models from Massive Datasets
According to researchers, the availability and diversity of data have a higher effect on the outcomes of machine learning models than the brilliance of the algorithm. In computers, it’s comparable to human experience.
As a result, expanding the dataset used to construct the predictive characteristics employed in a machine learning model may improve prediction accuracy.
When it comes to fraud detection, a model will benefit from the experience gained through consuming millions or billions of occurrences, both genuine and fraudulent transactions.
Superior fraud detection is achieved by analyzing a vast quantity of transactional data in order to better understand and predict risk on a per-person basis.
The application of behavioral analytics
In behavioral analytics, machine learning is used to assess and predict behavior across all parts of a transaction at a granular level. The data is organized into profiles that define each user’s, merchant’s, account’s, and device’s behaviors.
Such profiles are continuously updated with every transaction, thus allowing to draft analytic aspects that offer inch-perfect future behavior projections.
These profiles can include monetary and non-monetary transactions. For example, a request to change your password or address, or issuing a duplicate card, etc., all fall under non-monetary transactions.
For example, monetary transaction data aids in the building of patterns that may reflect a person’s usual spending velocity, the days and hours when they prefer to trade, and the time interval between geographically distributed payment venues, to name a few examples.
Profiles are particularly important since they give a current picture of activity, which can assist reduce transaction abandonment due to irritating false positives.
A good corporate fraud solution will include a number of analytic models and profiles that will offer the data needed to assess real-time transaction trends.
Deploying supervised and unsupervised AI models
Because organized crime strategies are so complex and versatile, defensive measures based on a single, one-size-fits-all analytic strategy will fail. Instead, each use case should be complemented with custom-built anomaly detection technologies that are best appropriate for the situation.
Since both the models are crucial while detecting fraud and should be integrated into next-generation fraud detection and prevention strategies.
The most prevalent type of machine learning in all domains, a supervised model, is one that is trained on a large number of precisely “labeled” transactions.
Each transaction is classified as either fraudulent or non-fraudulent. The models are trained by ingesting massive amounts of labeled transaction data in order to uncover patterns that best depict legal activity.
Model accuracy is intimately linked to the amount of clean, relevant training data utilized in the construction of a supervised model.
When labeled transaction data is sparse or non-existent, unsupervised models are used to detect unexpected behavior. Self-learning must be utilized in these cases to identify patterns in the data that standard analytics have missed.
Adaptive Analytics with Self-Learning AI
Fraudsters make securing consumers’ accounts extremely tough and dynamic, which is where machine learning shines. For continual performance improvement, fraud detection professionals should investigate adaptive systems that strengthen responses, particularly on marginal judgments.
These are transactions that are on the cusp of triggering the investigation, either slightly above or below the threshold.
The thin border between a false positive event — a legitimate transaction that scored too high — and a false negative event — a fraudulent transaction that scored too low — is where accuracy is most critical.
Adaptive analytics accentuates this distinction by offering a current awareness of a company’s risk factors.
Adaptive analytics solutions boost sensitivity to emerging fraud patterns by automatically responding to newly established case dispositions, resulting in a more accurate distinction between frauds and non-frauds.
When an analyst analyses a transaction, the outcome is given back into the system, whether the transaction is confirmed as valid or fraudulent.
This enables analysts to appropriately represent the fraud environment they are working with, including novel approaches and long-dormant fraud practices. In addition, changes to the model are made automatically with this adaptive modeling technique.
Using this adaptive modeling strategy, the weights of predictive parameters in the underlying fraud models are automatically updated. It’s an effective method for detecting fraud at the margins and blocking new types of fraud attacks.
Why employ AI for fraud detection?
Artificial intelligence helps to analyze bulk data to examine fraud patterns that can be utilized to detect real-time frauds.
When fraud is suspected, AI models may be used to either reject or flag transactions for additional investigation, as well as grade the probabilities of fraud, permitting investors to focus their efforts on the most promising cases.
Businesses have benefited from using AI to identify fraud by enhancing internal security and streamlining corporate procedures. As a result of its enhanced efficiency, Artificial Intelligence has emerged as a crucial instrument for preventing financial crimes.
The AI model can also provide cause codes for the flagged transaction. These reason codes instruct the investigator on where to look for flaws and help to expedite the inquiry.
When investigators review and clear problematic transactions, AI may learn from them, boosting the AI model’s expertise and avoiding tendencies that don’t lead to fraud.
Check out for AI certification courses if you want to pursue a career in artificial intelligence.
Final thoughts
The Banking and Retail industries are under attack and face numerous fraud charges as long as the modern world is flooded with card-not-present transactions conducted online. Email phishing, money fraud, identity theft, document forgery, and false accounts are all examples of criminal attacks on susceptible users’ data that result in data breaches.
New top-notch approaches based on Machine Learning algorithms for fraud detection and prevention are offering increasing value to organizations with their real-time work, speed, and efficiency while previous rule-based algorithms fade away.
If you are planning to becoming a cyber security engineer, then Blockchain Council’s extensive certification programs can be helpful to you. You can check out for cyber security training online courses or other programs according to your preferences. The courses offered at Blockchain Council are up to date and pocket-friendly too.