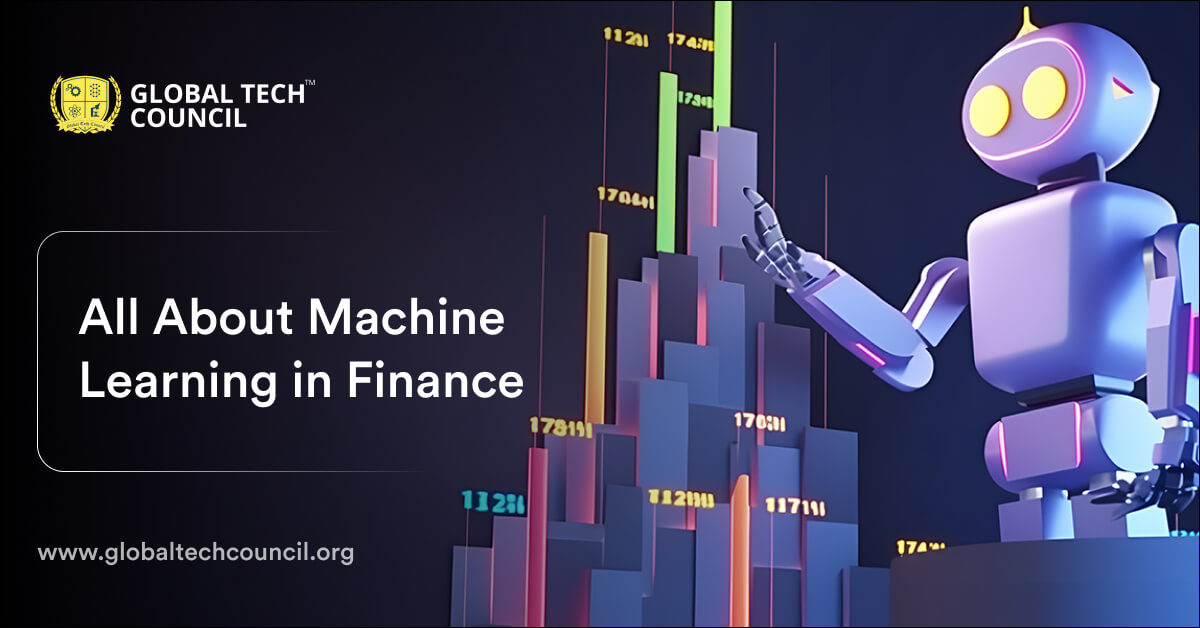
Summary
- Machine learning (ML) has revolutionized complex task execution, and businesses across various industries are embracing ML-based systems to streamline and optimize their processes.
- The finance industry can be transformed entirely by employing machine learning efficiently, and it has become a strategic imperative for financial organizations to stay competitive and meet customer expectations.
- ML is a specialized field within data science that leverages statistical models to extract insights and make predictions.
- AI and ML have a profound impact on optimizing internal operations and enhancing customer experiences, with a majority of customers comfortable handling their issues without human agents.
- ML is used in finance for fraud detection, risk management, process automation, data analytics, customer support, and algorithmic trading.
- Significant investments have been made in technology, such as general ledger technology and workflow automation, with the goal of achieving a touchless financial close by 2025.
- ML brings benefits such as minimizing human errors, time efficiency, cost effectiveness, workload reduction, and unbiased decision-making in the finance industry.
- ML applications in finance include algorithmic trading, fraud detection and prevention, portfolio management (robo-advisors), loan underwriting, investment predictions, process automation, secure transactions, mitigating risky transactions, financial advisory, customer data management, decision-making, customer service level improvement, customer retention programs, and marketing.
- Case studies highlight the successful implementation of ML in finance by companies such as Capital One, Sberbank, Revoleto, Credit Suisse, and Barclays.
- Challenges in implementing ML in finance include data privacy and security, ethical considerations, model interpretability, regulatory compliance, data quality and availability, and the need for a skilled workforce.
- ML has the potential to transform the finance industry, but addressing these challenges is crucial for successful implementation and adoption.
Over the past two decades, machine learning (ML) has emerged as a powerful and transformative force, revolutionizing complex tasks that were once limited to human experts. Today, businesses in various industries are embracing ML-based computerized systems to streamline and optimize their processes.
The finance industry, being one of the largest in the world, stands to benefit greatly from the efficient employment of machine learning. Embracing ML technology has become a trend and a strategic imperative for financial organizations aiming to stay competitive and meet the evolving expectations of their customers in the digital age. In this article, we will delve into how ML transforms finance and how you can reap its benefits.
Understanding Machine Learning
Machine Learning is a specialized field within data science that leverages statistical models to extract insights and make predictions. In this post, we will focus on the significance of machine learning.
Recent studies have emphasized the profound impact of Artificial Intelligence (AI) and ML in optimizing internal operations and enhancing customer experiences. Surprisingly, 65% of customers are comfortable resolving their issues without human agents, while an astonishing 74% prefer engaging with chatbots for simple questions.
The surge in ML adoption is driven by two key factors. Firstly, chatbots have been widely adopted, with over 60% of customers relying on them for assistance each year. Secondly, chatbots have proven to be highly cost-effective, saving an estimated $1.3 trillion annually for eCommerce businesses by efficiently addressing customer requests.
Why is Machine Learning Used in Finance?
Machine learning applications in the finance industry have gained widespread adoption, driving advancements in fraud detection, risk management, process automation, data analytics, customer support, and algorithmic trading. As technology continues to evolve, the goal is to achieve autonomous finance using ML, AI, and influential technologies like Blockchain.
A recent survey conducted by Gartner in 2022 revealed that significant investments were made in technology, such as general ledger technology, immediate financial solutions, and workflow automation. These technologies have already been successfully implemented in over half of the finance functions. Executives set an ambitious target for a touchless financial close by 2025, envisioning the complete automation of the immediate financial process, eliminating the need for human intervention.
The finance industry recognizes the potential of artificial intelligence and machine learning to streamline operations, enhance efficiency, and drive innovation. Embracing these technologies has become a strategic imperative for businesses looking to stay ahead of the competition, leading to an increase in the hiring of individuals with a finance background who can implement ML models into their operations.
Benefits of Machine Learning in Finance
The integration of machine learning in finance brings forth numerous benefits, contributing to the advancement of the industry. Let’s explore some of these advantages in detail:
- Minimization of Human Errors: Machine learning algorithms ensure accurate data processing and decision-making, significantly reducing human errors that could lead to substantial financial losses.
- Time Efficiency: ML enables real-time solutions and expedites manual processes, allowing financial professionals to make complex decisions swiftly and generate accurate predictions, saving valuable time.
- Cost Effectiveness: Advances in AI and machine learning technologies improve their efficacy and efficiency over time, leading to cost savings and improved financial operations.
- Workload Reduction: Machine learning algorithms excel at handling complex, repetitive, and time-consuming tasks, freeing up finance professionals to focus on higher-value activities and strategic decision-making.
- Unbiased Decision-Making: ML algorithms make data-driven decisions without inherent biases or preconceived notions, ensuring fair and transparent judgment, which is crucial in the finance industry.
Applications of Machine Learning in Finance
Having understood the reasons for using ML in finance and its associated benefits, let’s explore how machine learning can be directly applied to financial functions in the industry:
- Algorithmic Trading: Machine learning algorithms analyze real-time business news and trade activities to make informed trading decisions, providing traders with a competitive edge over conventional methods. Algorithmic trading enhances market efficiency by eliminating emotional biases.
- Fraud Detection and Prevention: Machine learning algorithms play a crucial role in detecting and preventing fraudulent activities. By analyzing large datasets, these algorithms identify anomalies or patterns indicative of fraud, flagging suspicious transactions for further investigation.
- Portfolio Management (Robo-advisors): Robo-advisors utilize machine learning algorithms to offer personalized financial advice to investors. By analyzing real-time market trends, these platforms construct optimized portfolios based on investors’ goals and risk preferences, providing cost-effective and accessible investment solutions.
- Loan Underwriting: Machine learning simplifies the loan underwriting process for banks and insurance companies by training algorithms on extensive consumer data. These algorithms analyze various factors to assess loan eligibility, reducing time and costs associated with manual underwriting.
- Investment Predictions: Machine learning enables fund managers to make informed investment decisions by analyzing market trends and identifying changes earlier than traditional models. Automated investment advisors powered by machine learning are disrupting the investment banking industry.
- Process Automation: Machine learning facilitates the automation of repetitive tasks, such as paperwork, customer support through chatbots, and employee training gamification. This improves customer experience, reduces costs, and enhances scalability.
- Secure Transactions: Machine learning algorithms excel at detecting transactional fraud by analyzing large volumes of data and identifying patterns that may go unnoticed by humans. Real-time fraud prevention and identification systems are being deployed to minimize losses.
- Mitigating Risky Transactions: Machine learning techniques help banks and financial institutions mitigate risk by analyzing vast data beyond traditional factors like credit scores. Insights derived from machine learning models assist in making informed decisions and predicting default risks.
- Financial Advisory: Machine learning powers budget management apps and robo-advisors that offer personalized financial advice and guidance based on individual spending patterns, helping users track spending, manage investments, and plan for retirement.
- Customer Data Management: Machine learning aids in processing and extracting insights from large volumes of financial data, enabling efficient data management and improved business profitability. Data analytics, mining, and natural language processing techniques improve decision-making.
- Decision-Making: Machine learning algorithms analyze structured and unstructured data to discover trends, assess risks, and support informed decision-making, utilizing customer requests, social media interactions, and internal business processes.
- Customer Service Level Improvement: Intelligent chatbots powered by machine learning enhance customer service by providing prompt and accurate responses to queries, helping customers with financial planning, loan eligibility, and other services.
- Customer Retention Programs: Machine learning algorithms predict customers at risk of attrition based on demographic data and transaction activity, enabling companies to design targeted retention offers and attract new customers with demographic-specific investment schemes.
- Marketing: Machine learning models leverage past behavior data to make accurate predictions and support marketing strategies, helping finance companies create effective campaigns and personalized offers.
These use cases demonstrate the transformative impact of machine learning on various aspects of the finance industry, enhancing security, risk management, customer experience, and data-driven decision-making. By adopting ML practices, the finance industry is set to undergo positive changes and increase consumers’ trust in financial services.
Case Studies
Let’s explore a few case studies that showcase how machine learning is effectively utilized in the finance industry:
- Capital One: Capital One employs machine learning algorithms to identify fraudulent credit card transactions. By analyzing factors such as transaction timing, amount, and location, these algorithms swiftly recognize suspicious activities, protecting customers and preventing financial losses.
- Sberbank: Russia’s largest bank, Sberbank, utilizes machine learning to predict customer spending patterns. By leveraging data-driven insights, they personalize their offers, resulting in increased sales and customer satisfaction.
- Revoleto: The e-learning platform Revoleto leverages machine learning to recommend investment courses tailored to users’ interests and goals. This personalized approach enables users to explore the world of investments with confidence and relevance.
- Credit Suisse: Credit Suisse utilizes machine learning algorithms to assess the risk of loan defaults. By integrating this technology into their lending decisions, they optimize risk management and ensure informed lending practices.
- Barclays: Barclays employs machine learning to detect fraudulent transactions, safeguarding their customers from financial losses. By continuously analyzing patterns and anomalies, Barclays reinforces trust and security.
Challenges of Machine Learning in Finance.
While machine learning offers numerous benefits to the finance industry, there are also challenges that need to be addressed:
- Data Privacy and Security: As financial data is highly sensitive, ensuring data privacy and security becomes crucial when implementing machine learning solutions.
- Ethical Considerations: Machine learning algorithms should be designed and trained to avoid biases and discriminatory practices to ensure fair and ethical decision-making.
- Model Interpretability: Interpreting complex machine learning models and understanding their decision-making processes can be challenging, which may limit their acceptance and adoption in certain situations.
- Regulatory Compliance: Financial institutions need to ensure that their machine-learning solutions comply with regulatory requirements and standards to maintain trust and transparency.
- Data Quality and Availability: Access to high-quality and relevant data is essential for training accurate and reliable machine learning models. Ensuring data availability and quality can be a significant challenge.
- Skilled Workforce: Developing and implementing machine learning solutions in finance requires a skilled workforce with expertise in both finance and machine learning. Acquiring and retaining such talent can be a challenge for organizations.
Conclusion
Machine learning has the potential to transform the finance industry, enabling automation, enhancing security, improving customer experiences, and driving data-driven decision-making. By leveraging machine learning applications such as algorithmic trading, fraud detection, portfolio management, and process automation, financial institutions can optimize their operations and stay ahead in the competitive landscape. However, it is crucial to address challenges related to data privacy, ethical considerations, interpretability, regulatory compliance, data quality, and skilled workforce to ensure the successful implementation and adoption of machine learning in finance.